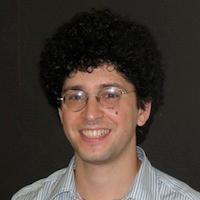
Jonathan Tannenhauser
Lecturer in Mathematics
Background in theoretical particle physics, focusing on a conjectured equivalence between certain quantum field theories and certain string theories.
Professor Tannenhauser's background is in theoretical particle physics, where his work has focused on the the AdS/CFT correspondence, a conjectured equivalence between certain quantum field theories and certain string theories. More recently he has become interested in applying computational and statistical tools to the genomics of birdsong. The goal is to pinpoint which genes are expressed in a singing bird's brain and how the expression pattern changes over the course of brain development.
Education
- B.A., Harvard University
- M.A., University of California-Berkeley
- Ph.D., University of California-Berkeley
Current and upcoming courses
Linear Algebra
MATH206
Linear algebra is one of the most beautiful subjects in the undergraduate mathematics curriculum. It is also one of the most important with many possible applications. In this course, students learn computational techniques that have widespread applications in the natural and social sciences as well as in industry, finance, and management. There is also a focus on learning how to understand and write mathematical proofs and an emphasis on improving mathematical style and sophistication. Topics include vector spaces, subspaces, linear independence, bases, dimension, inner products, linear transformations, matrix representations, range and null spaces, inverses, and eigenvalues.
-
Multivariable Calculus
MATH205
Most real-world systems that one may want to model, whether in the natural or in the social sciences, have many interdependent parameters. To apply calculus to these systems, we need to extend the ideas and techniques of single-variable Calculus to functions of more than one variable. Topics include vectors, matrices, determinants, polar, cylindrical, and spherical coordinates, curves, partial derivatives, gradients and directional derivatives, Lagrange multipliers, multiple integrals, vector calculus: line integrals, surface integrals, divergence, curl, Green's Theorem, Divergence Theorem, and Stokes’ Theorem.