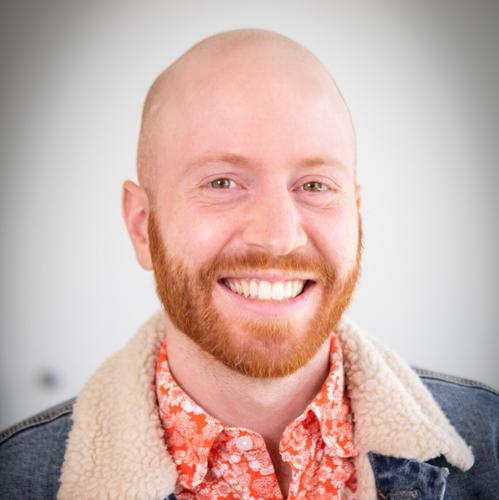
Yaniv Yacoby
Assistant Professor of Computer Science
Links
I'm an interdisciplinary researcher working at the intersection of machine learning (ML) and mental health.
I lead the Model-Guided Uncertainty (MOGU) Lab at Wellesley, where we enable effective and responsible uses of expressive (deep) ML models in safety-critical domains, like precision healthcare. Our research specifically focuses on developing methods to help us better understand, predict, and prevent suicide and related behaviors. We do this by developing new paradigms for clinician/patient-AI collaboration.
I see both my teaching and research as social endeavors. My teaching and research both require a social context to meaningfully center ethics, and require supportive classroom/lab cultures to support holistic growth. I'm therefore excited about creating classroom and mentorship experiences that emphasize community building and interrogation of socio-technical systems and cultures.
Outside of work, I enjoy spending time with my two cats and dog, playing and listening to folk music, trying out new food, and watching reality TV.
For my CV, as well as information about my research, teaching, and service, see my website.
Education
- B.A., Harvard University
- M.M., New England Conservatory of Music
- Ph.D., Harvard University
Current and upcoming courses
Data Structures
CS230
An introduction to techniques and building blocks for organizing large programs. Topics include: modules, abstract data types, recursion, algorithmic efficiency, and the use and implementation of standard data structures and algorithms, such as lists, trees, graphs, stacks, queues, priority queues, tables, sorting, and searching. Students become familiar with these concepts through weekly programming assignments using the Java programming language.
A student is required to have confirmation of authorization from the CS 111 faculty that is based on the mastery of the CS 111 concepts in order to enroll in CS 230. Students who did not take CS 111 at Wellesley and who wish to enroll in CS 230 should contact the CS department to take a placement questionnaire.
During the initial registration period (November 2024), students with a recommendation from CS 111 faculty will be prioritized and allowed to register. If you do not fall in that category and would like to enroll in CS 230 during Spring 2025, please fill out this form, as registration after the initial period will be by Permission of Instructor (POI) only. Filling out this form indicates student interest to enroll; it does not necessarily guarantee a seat in the course.