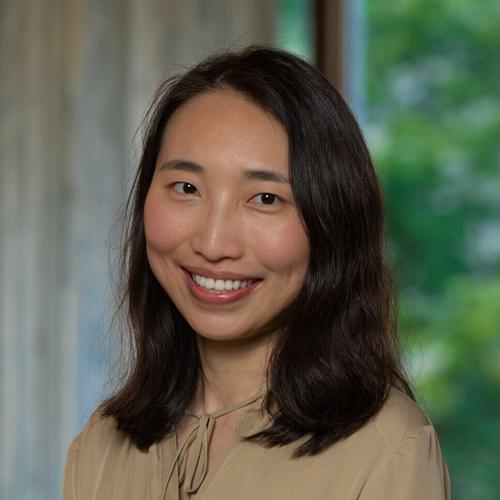
Qing (Wendy) Wang
Associate Professor of Mathematics
Links
Statistician specializing in topics of U-statistics, nonparametric kernel density estimation, risk estimation, variance estimation, cross-validation, resampling schemes, and extrapolation/interpolation methods.
I was born and raised in Beijing, China. I came to the U.S. to pursue a graduate degree in 2007, and received a Ph.D. in Statistics from Penn State University in 2012 under the supervision of the late Dr. Bruce G. Lindsay. Prior to joining Wellesley, I was a tenure-track Assistant Professor of Statistics at Williams College from 2012 to 2015, and at Bentley University from 2015 to 2016.
My research focus on topics in U-statistics, risk estimation, variance estimation, and cross-validation. Some of my recent research interests also include penalization methods, model assessment tools, mediation analysis and jackknife empirical likelihood. My teaching considers both applied and theoretical topics in statistics, including introductory statistics, regression analysis, probability, multivariate data analysis, nonparametric statistics, and Bayesian statistics. I greatly enjoy working with students on research topics in statistics. I also have great passion in undergraduate statistics education, and I am interested in incorporating active learning and creating interactive and hands-on educational tools for teaching statistics.
I am one of the advisors for the new data science major at Wellesley. To learn more about the data science major requirements, please visit this website. (Note that effective Fall 2023, data science is no longer a structured individual major, but a regular major. Please refer to the data science website for the current DS major requirements.)
In my spare time, I enjoy baking, cooking, practicing yoga, traveling with my husband, and spending time with my two young children, Iwa and Ian.
Education
- B.S., Beijing Normal University (北京师范大学)
- M.S., Pennsylvania State University-Main Campus
- Ph.D., Pennsylvania State University-Main Campus
Current and upcoming courses
Capstone in Data Science
DS340H
-
Introductory Statistics and Data Analysis
STAT218
This is a calculus-based introductory statistics course. Topics covered include data collection, data visualization, descriptive statistics, linear regression, sampling schemes, design of experiment, probability, random variables (both discrete and continuous cases), Normal model, statistical tests and inference (e.g. one-sample and two-sample z-tests and t-tests, chi-square test, etc). Statistical language R will be used throughout the course to realize data visualization, linear regression, simulations, and statistical tests and inference. -
Multivariate Data Analysis
STAT228
This is a course in multivariate data analysis. Students will be introduced to modern multivariate techniques, their applications and interpretations, and will learn how to use these methods to understand relationships between variables, extract patterns, or identify clusters or classifications in a rich data set involving multiple variables. Topics covered during the semester include both dependence techniques (e.g. multiple linear regression, binary logistic regression, multinomial logistic regression, principal component analysis, linear discriminant analysis, decision trees, etc) and interdependence techniques (e.g. factor analysis, cluster analysis, etc). A selection of topics in machine learning and data mining are also introduced during the semester. Statistical language R is used in this class.